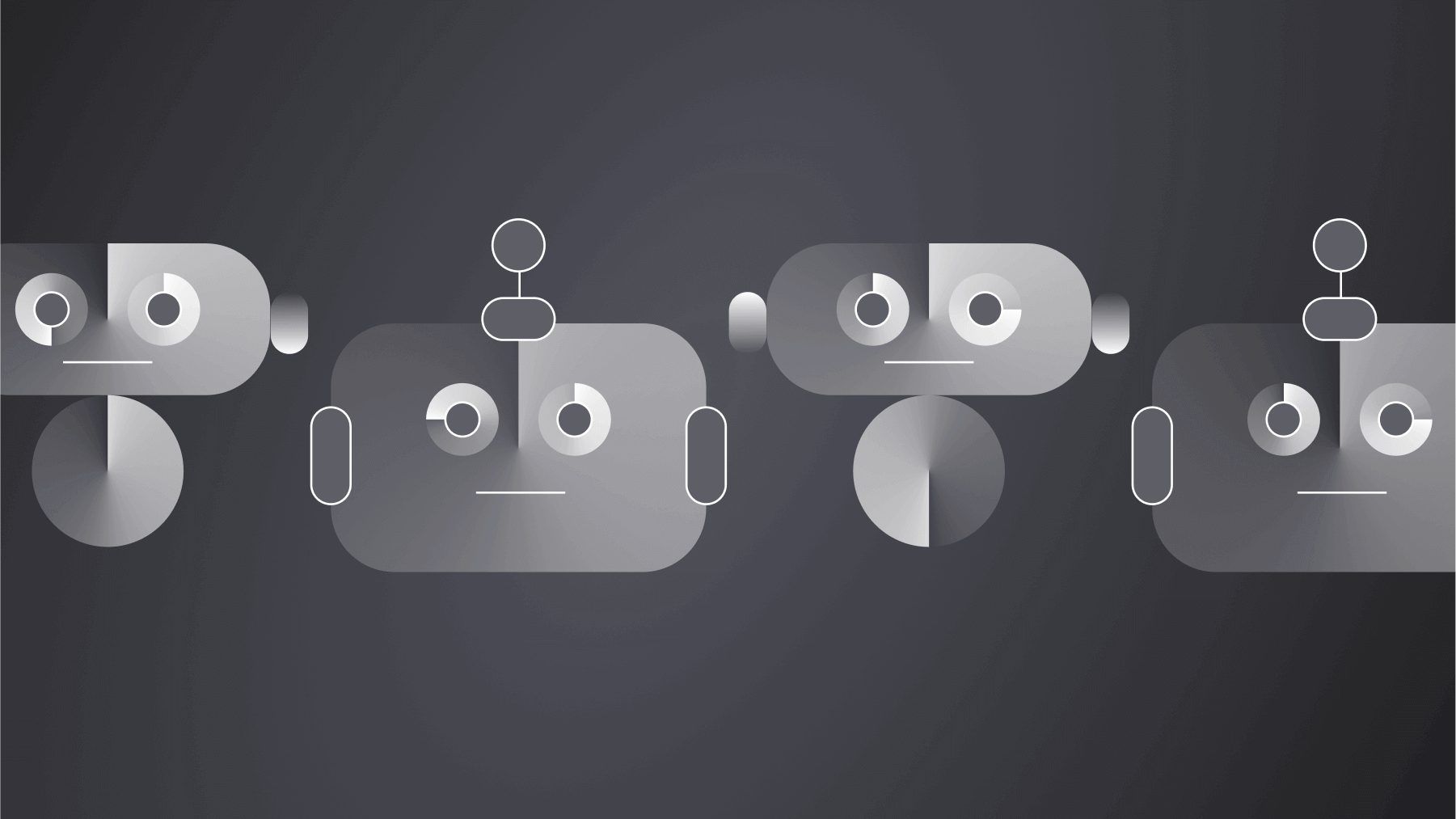
In 2020, over one-third of all Internet users were bots, according to a study by Imperva.
While there are many "good bots," such as those that index content for search engines, help to schedule appointments, or automate customer service, there are also "bad bots." These malicious bots are responsible for everything from spam and identity theft to denial-of-service attacks and click fraud.
The problem of bots reaches into all industries online. In 2021, most companies (83%) surveyed experienced at least one bot attack in the last year, resulting in loss of revenue and increased operational costs. Of those, 77% lost 6% or more of revenue due to bot attacks, and 39% reported a revenue loss of 10% or more.
In this guide, we'll explore what bots are, how they’re used, the challenges in detection and how businesses can protect against them through bot detection software.
What are bots, and how are they used?
Bots are simple computer programs that automate tasks. For example, on the bottom-right of this page, you can see a chatbot icon. That's a bot. For this guide, we're referring to malicious bots that flood the internet rather than helpful ones.
Spam
Bots have overrun the "public square" of the internet, and their spammy behavior worsens the signal-to-noise ratio. Spam is unsolicited, irrelevant, or low-quality content posted to clutter a website or online forum. It is a form of digital pollution that can make it difficult for users to find the information they want.
Bots can create spam content at a much higher rate than humans, often using stolen or fake accounts, making it hard for platform owners to moderate their content, making it more likely that users will see spammy content in their feeds.
Social media platforms use algorithms to surface content compounding the problem of bot-generation spam. These algorithms maximize engagement by showing users relevant content likely to generate likes, comments, and shares. However, spammers use these algorithms to create content designed to be clicked on and shared. This "click bait" can spread rapidly through social networks, clogging up users' feeds with low-quality content.
These bots work by creating large numbers of social media accounts and using them to follow, like, and comment on other users' content. The bots are programmed to leave comments designed to entice users to click on links, often leading to spammy or low-quality websites.
Bots can be very effective at spreading spam because they often have thousands of followers and generate many likes and comments on the content they share, making it more likely that other users will see and potentially click on the links.
It’s important to note that social media isn’t alone when it comes to businesses affected by spam. Spam happens within an unprotected form, with programmatic fake reviews on product pages and more.
DDoS attacks
Bots also assist in launching DDoS attacks, offering more automation over traditional attack methods. For example, bots can be easily controlled and commanded to carry out an attack, quickly generating a large amount of traffic.
One standard attack method is using botnets, which are networks of infected computers that the attacker can control. The attacker can then use these computers to generate vast amounts of traffic, making it difficult for the target website or server to cope.
Another standard method is a reflector attack, which involves flooding a target with UDP packets reflected by a server, amplifying the target's traffic, making coping even more challenging.
IT services downtime costs companies from $300,000 to over $1 million per hour, so even a short DDoS attack can have a significant financial impact.
Well-resourced attackers can use a botnet of tens of thousands of computers to generate massive traffic, making it difficult for the target website or server to cope. For example, botnets targeted thousands of Google's IP addresses over six months in 2020, where the attacker spoofed 167 million packets per second, peaking at 2.5 terabytes per second.
While Google's security engineers were eventually able to mitigate the attack, it highlights the scale of bot-powered DDoS attacks that are now possible. Unfortunately, DNS provider Dyn wasn't so lucky, and amidst a 1 TBPS attack in 2016, several high-profile sites, including Twitter, Reddit, PayPal, Netflix, and Airbnb, were knocked offline.
Fake engagement
The most common social media bots create fake accounts or "sock puppets." These sock puppet accounts are then used to like, comment on, and share content from the botmaster's real account to increase their visibility and engagement. Marketers often use bots to boost their social media presence or inflate their clients' engagement statistics.
For example, a bot could automatically "like" every tweet that includes a specific hashtag on Twitter, artificially inflating that hashtag's apparent popularity and making it more likely to trend. Similarly, botnets (multiple bots acting together) can boost the follower count of an account to make it appear more popular than it is.
Because of this, some public discourse on social media isn't an accurate representation of reality. For example, when bots artificially inflate the content's popularity, it can give a false impression that a particular topic is far more critical or exciting than it is.
In reality, bots distort actual human attention, opinions, and sentiment, resulting in far-reaching effects. The results of this distortion include skewing public opinion and distorting the reach of important news stories, which extend beyond inflating social media followers and creating a faux "influencer" ecosystem.
Some marketing agencies and influencers use bots to generate fake "buzz" around their products or services. Unfortunately, executives unaware of what's happening fall prey to vanity metrics and make decisions based on false information. Influencer marketing fraud costs companies a shocking $1.3 billion annually. According to research by HypeAuditor, many prominent influences are involved in fraudulent activity, including buying likes and followers.
Source: HypeAuditor State of Influencer Marketing Report
When bots create fake engagement, trust erodes in social media platforms and brands. For example, suppose people can't trust that the conversations they're seeing are honest or that the popularity of a product is genuine. In that case, social media loses its value as a platform for connection and communication.
Social media analytics platforms highlight the view counts of various topics but don't show how many views come from real people or bots. This lack of transparency makes it difficult to detect fake engagement and leads to a distorted understanding of what's popular on social media.
As bots become more sophisticated, it will only become more difficult to discern real engagement from fake. As a result, businesses and individuals using bots for gaming the system ultimately damage the social media ecosystem for everyone.
Spreading malware
According to a report by Sitelock, a leading website security company, malware almost doubled last year, much of which is spread by bots.
Bots can be used to create fake accounts on social media platforms and review sites and then post links to malicious websites. These links can spread quickly through networks of friends and followers, infecting hundreds or even thousands of computers.
Bots can also be used to create fake accounts on search engines and then post links to malicious websites. When people search for specific keywords, these links will come up in the results, and unsuspecting users may click on them, thinking they're going to a legitimate website. But instead, they'll end up on a site that tries to install malware on their computer.
These malware attacks can inject malicious code into a user's browser that scammers use to steal personal information or hijack the victim's computer for purposes such as account takeover to demanding ransom payments.
Attackers can sell stolen accounts, engage in identity theft, take over a user's machine to mine cryptocurrency, or use it as a bot in a botnet. Some are targeted attacks, while others are part of a much larger campaign. For example, the 2016 Mirai botnet attack that took down parts of the internet involved over 600,000 IoT devices infected with malware.
The Mirai botnet reemerged this year, and there's been a rise in botnet activity in the first quarter of 2022. Another notorious botnet, Emotet, called "the most dangerous malware in the world" by Europol, has seen a growth of over 200% in March 2022.
This problem is only going to get worse as bots become more sophisticated. And it's not just social media platforms and search engines at risk. Any website that allows users to post links could be vulnerable to these attacks.
Phishing attacks
Phishing attacks are on the rise, and bots are to blame. During the pandemic, phishing incidents rose by 220%. Several pandemic-related drivers for this growth include more lax security standards in work-from-home environments, increased anxiety and stress, the prevalence of bring-your-own-device (BYOD) policies, and even more time on devices and in front of screens.
Social media platforms are teeming with bots capable of launching programmed phishing attacks. Unfortunately, these attacks are becoming more sophisticated and harder to detect.
Phishing is a cyber attack that uses email or malicious websites to trick users into providing personal information, such as login credentials or credit card numbers. The attackers then use this information to access the victim's accounts or financial data. Phishing attacks can also spread malware or ransomware.
Bots can automate many steps in a phishing attack. For example, a bot can create fake social media profiles and populate them with personal information scraped from the internet. The bot can then use these profiles to a friend or follow victims. Once the victim's friend follows the bot's fake profile, the bot can send them direct messages that contain links to malicious websites or attachments infected with malware.
Consider a programmed Twitter bot that launches phishing attacks. First, the bot can create a fake Twitter profile and start following people. Then, it sends direct messages to all of the account’s followers. The DMs contain links to malicious websites that look like legitimate websites.
When victims click on the link, they end up on a website that looks identical to the actual website. However, the URL of the website is slightly different. For example, instead of twitter.com, the URL might be tw1tter.com or t3itter.com. This trick is known as typosquatting, often used in phishing attacks.
Once victims are on the fake website, they are prompted to enter their login credentials or credit card information. Once they enter this information, they also send it to the attacker, who can use it to access the victim's account or financial data.
Or, on LinkedIn, attackers may automatically use bots to add connections with as many people as possible. LinkedIn's search feature makes it easy to find people with specific job titles or who work for particular companies. Once the attacker has added a victim as a connection, they can send them a direct message. The message may contain a link to a malicious website or an infected attachment with malware.
Even on Tinder, there are many potential types of phishing attacks. For example, an attacker could create a fake profile and start swiping right on as many people as possible. Once a match succeeds, the attacker could begin chatting with the victim and try to get them to click on a link to a malicious website. The attacker could even bring the victim to another platform, like Snapchat or WhatsApp, to bypass Tinder's security features.
Bots are becoming more sophisticated and challenging to detect. Bots can imitate human behavior, such as liking and sharing or even generating content, making it more difficult for victims to tell if they are interacting with a real person or a bot. Attackers can also use bots to create fake reviews on websites. These fake reviews can trick victims into thinking that a website is safe when it is not.
The cost of phishing attacks can be high. In 2021, the average cost of a phishing attack was a whopping $3.2 million. This cost includes the loss of productivity, the cost of investigating and responding to the attack, and the damage to the brand's reputation. In some cases, the costs can be much higher.
The challenges of bot detection
Bot detection is challenging because they often employ tactics such as using proxy servers or changing their IP address frequently, making it challenging to track down their location or identity. Furthermore, many bot creators use multiple accounts to spread their bot's activity across different platforms to evade detection. And there are far too many bots for manual detection to be feasible.
A report from the Pew Research Center found that automated accounts – not human beings post two-thirds of tweeted links to popular websites. With such a large number of bots active on social media platforms, it becomes challenging to identify them.
Another reason it is challenging to detect bots is that they often mimic human behavior. For example, a Twitter bot might follow many users and retweet their content to appear like a real person. Many bot creators even go so far as to create fake profiles with photos and biographies that make them seem like real people.
Not only that, but the costs of false positives – an actual user mistakenly identified as a bot – are high. If that occurs, users might be suspended or banned from platforms if they are incorrectly flagged as bots, leading to a loss of revenue for businesses that rely on social media to reach their customers.
The problem of detecting bots is further complicated by the fact that there is a large gray area between what is considered a bot and what is considered a legitimate use of automation technologies. For example, many programs allow users to automate their social media activity. These programs are not necessarily evil or malicious, but they can be used to create bot-like behavior.
In light of all these challenges, it is clear that detecting bots is difficult. Bot creators are constantly evolving their tactics to evade detection, and it is becoming increasingly difficult to tell the difference between a real person and a bot. However, as businesses become more aware of the problem, they are beginning to invest in solutions that can help to identify and track down bots.
Why Legacy Approaches to Bot Detection Fail
One of the most common methods for detecting bots is looking for simple heuristics or behavior patterns indicative of automated activity. For example, a bot might post more often than a human user or use different words than a human would.
However, modern bots avoid these types of heuristics. For example, they might post less often than a human user or use synonyms for common words to avoid flagging. As a result, heuristics-based detection methods are no longer effective at identifying bots.
In another example, a rule might say that any account that posts more than 50 times per day is a bot. However, bot creators easily circumvent these types of practices. For example, they could divide their bot's activity into multiple accounts that each post fewer than 50 times per day.
Another commonly used method for detecting bots is to analyze IP addresses by looking at the number of accounts registered from a single IP address or the location of the IP address (if it is known). However, this method is not foolproof because many bots now use IP address rotation, which means they change their IP address frequently to avoid detection. In addition, some bot makers host their bots on servers in different locations worldwide, making it challenging to identify the bot's source.
As you can see, traditional methods for bot detection are no longer effective. This is because bot makers have become quite skilled at evading detection. As a result, these methods can no longer identify bots to the accuracy required to prevent bot attacks effectively.
How Fingerprint solves the bot problem
BotD is an open-source JavaScript bot detection library that uses fingerprinting to identify bots accurately in real-time. Built on the foundation of Fingerprint’s device identification platform, BotD utilizes hundreds of signals from browsers and devices to accurately determine if a “user” is a malicious bot, a harmless crawler, or a human.
In addition, it provides complete transparency into what data is collected, meaning you can be sure there's no shared personal information. The library has four valuable detectors: automation tools, search engines, browser spoofing, and virtual machine detection.
Our bot detection solution provides a singular response with three available parameters, which we define a bit more below:
- "Good" if the bot is a search engine or friendly crawler
- "Bad" if the bot is an automated tool or virtual machine
- "Not Detected" if the visitor is not considered a bot.
BotD addresses two groups of “traffic” in its detection process, general invalid traffic (GIVT) and sophisticated invalid traffic (SIVT).
General Invalid Traffic (GIVT)
This type of traffic is not meant to be harmful but is still considered to be a bot or non-human. GIVT typically consists of web crawlers from large tech companies, like Google Search and advertising bots. This type of traffic does not attempt to simulate human behaviors.
While businesses need to detect bad bots, it's essential to ensure that good bots can crawl unhindered. For example, BotD can identify many search engine bots, including Google Bot, Bing Bot, DuckDuckGo Bot, and many more, from social media bots to web crawlers.
Sophisticated Invalid Traffic (SIVT)
Sophisticated invalid traffic is more difficult to detect, hence the name. SIVT classifies bots that attempt to replicate human behavior and try malicious activities. SIVT includes a broad range of automated tools, botnets, headless and browsers like Selenium, Geb, CasperJS, and many more.
Bad actors on the internet are constantly devising new ways to run fraudulent schemes with bots but no matter how sophisticated the bad actor, BotD will be able to detect their presence and thwart their efforts.
The bottom line
There is ever-increasing bot activity on social media platforms and websites. They are used to defraud marketing & advertising spends, spread malware, enact phishing attacks, distort public opinion, hamper the customer experience, and more.
The problem will only worsen as bots become more sophisticated and challenging to detect. In addition, traditional methods for bot detection, which rely on simple heuristics, are no longer effective in an age where attacks are spoofing devices and IP addresses.
The costs of inaction are high. For businesses, the risks include loss of revenue, brand damage, and decreased customer trust. For society, the hazards include manipulation of public opinion, increased polarization, and erosion of trust in institutions. The modern world is built on trust, and bots erode that trust.
This is why we built BotD – to provide an accurate, transparent, and easy-to-use bot detection solution. BotD uses fingerprinting to identify bots in real-time, meaning that you can be sure that the data you're seeing is coming from real people.
If you suspect bots are impacting your business, we urge you to take action. Implementing BotD is one of the best things you can do to protect your business from the damaging effects of bots.